How to not fail with generative AI projects!
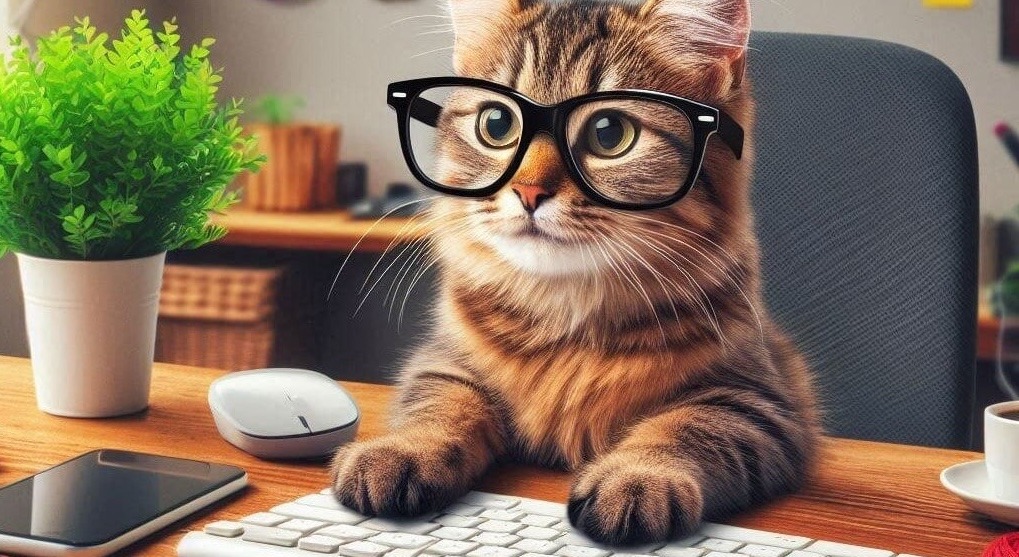
In the thrilling race to utilize the power of generative AI, companies around the world are jumping on the bandwagon faster than you can say “artificial intelligence.” But, as the saying goes, "Not all that glitters is gold"—and not all AI projects are destined for glory. In fact, according to a recent article from Computer Sweden, nearly a third of these shiny new AI projects might end up in the digital dumpster. Ouch.
But fear not! If you want your generative AI initiative to be more than just a cautionary tale at the next industry conference, there's a secret weapon you absolutely need: software quality assurance (SQA). Sure, SQA might not sound as glamorous as the latest AI buzzwords, but trust me, it’s the hidden gem that can save your project from going belly up.
The High-Stakes Game of Generative AI
Generative AI, with its magical ability to create everything from convincing chatbots to AI-generated cat memes, is the talk of the town. By 2026, Gartner Research predicts that 80% of companies will have adopted generative AI in some form. Yet, before you start dreaming of AI-powered utopias, remember that about a third of these projects will likely be abandoned faster than a New Year’s resolution.
Why, you ask? Well, it turns out that generative AI is a bit like a high-maintenance pet: it requires constant attention, can cost you a small fortune, and might just leave you questioning your life choices. Poor data quality, runaway costs, and unclear business value are just a few of the pitfalls that have sent many an AI project to the scrap heap.
Why SQA is Your AI Project’s Best Friend
Enter SQA, the trusty sidekick every AI project needs but rarely gets the credit it deserves. Here’s how SQA can help you avoid the dreaded “AI project fail” label:
-
Data Integrity: The Backbone of Success
Imagine trying to train a dog using conflicting commands. Now imagine doing the same with an AI model using poor-quality data—chaos ensues. Ensuring your AI is trained on high-quality data is crucial, but it’s not always easy or cheap. While collecting good data is a big investment, checking its quality through SQA can be equally challenging, given the volume and complexity involved.However, by using synthetic and augmented data generated from clean sources, you can stretch your data’s value without breaking the bank. Many AI models already use this strategy to enhance training while keeping costs manageable. Of course, even with synthetic data, solid QA is essential to maintain integrity and set your project up for success.
-
Risk Management: Because Nobody Likes Surprises
AI projects can be like a box of chocolates—you never know what you're going to get. That is, unless you have solid risk controls in place. SQA helps identify potential issues before they become full-blown disasters, keeping your AI project on the right track and free from nasty surprises (like data breaches or rogue algorithms). -
Cost Control: Keeping Your AI Budget in Check
Building a generative AI system without SQA is like throwing money into a black hole and hoping something useful comes out the other side. Spoiler alert: it usually doesn’t. SQA helps you catch inefficiencies early, so you’re not left wondering why your AI budget has mysteriously doubled overnight. -
Proving the ROI: The Final Frontier
Ah, ROI—the elusive pot of gold at the end of the AI rainbow. Everyone wants it, but few can find it. With SQA, you have a much better shot at proving your AI investment was worth it. By ensuring your AI systems are aligned with business goals, SQA helps you demonstrate tangible value, rather than leaving you mumbling excuses at the next board meeting.
Other Key Ingredients for AI Success
Now, let’s be real—SQA isn’t a magic wand that will make all your AI dreams come true (though it gets pretty close!). You’ll also need a few other key ingredients to ensure success:
-
Patience and Persistence: Remember, Rome wasn’t built in a day, and neither is a functional AI model. Be ready to iterate, tweak, and, yes, fail a few times before you hit the jackpot.
-
A Sense of Humor: Trust me, you’ll need it when your AI starts spitting out answers that are, well, creative. Embrace the weirdness—it’s all part of the journey.
-
Cross-Functional Collaboration: AI projects are like team sports—you need all the players (data scientists, engineers, QA folks, and business stakeholders) on the same page if you want to score.
Conclusion: AI Success is a Marathon, Not a Sprint
So, there you have it—a brief guide on how to succeed with generative AI projects. While quality assurance, particularly for data, may not be the most glamorous aspect of AI development, it is undoubtedly one of the most crucial. By combining it with realism, collaboration, and a touch of humor, you are well on your way to making your AI ambitions a reality.
The importance of thorough quality assurance is further underscored by industry insights, such as those highlighted in the NIST article on AI assurance. Achieving consensus on AI assurance practices is critical for ensuring that AI systems are reliable, safe, and effective. This path to consensus emphasizes how essential QA is for any AI project that aims to deliver lasting value.
Remember, in the world of AI, it’s not just about being the fastest or the first—it’s about being the one who actually gets it right. And with SQA by your side, you’ll have a much better chance of crossing the finish line in style.
Want to learn more about SQA and AI? Read more here, or contact us directly.