Navigating the AI Frontier: Elevating QA for AI Systems
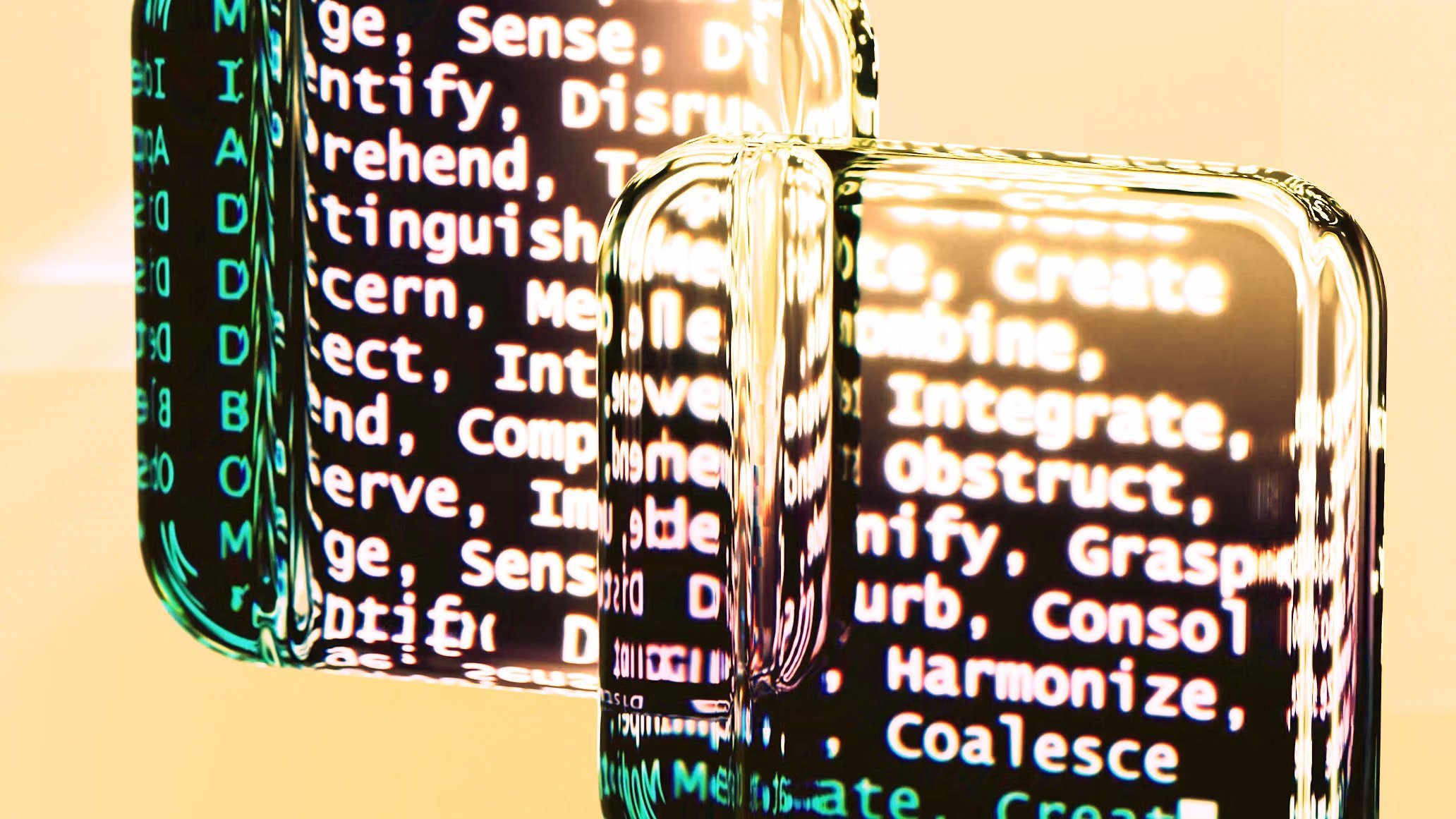
As someone who transitioned from software development and data science into the world of quality assurance (QA), my first weeks at System Verification were a whirlwind of discovery. While my expertise in machine learning (ML) and MLOps was warmly welcomed, I quickly realized I had to learn more about QA and testing. It was a steep learning curve, but the eagerness of my colleagues for technical insights gave me a clear mission: to bridge the gap between our strong QA foundation and the rapidly evolving AI landscape.
Defining the Market for AI in QA
When I started, one thing was certain: I was here to educate my peers on ML, data engineering, and MLOps. However, it wasn’t immediately clear how these areas aligned with our core mission of providing high-quality QA services. Through countless conversations with colleagues and customers, and a deep dive into industry trends, two opportunities emerged.
First, QA in AI-based systems is largely an untapped field. AI teams often rush from prototype to prototype without fully testing data pipelines, models, or supporting applications. This lack of proper QA makes AI systems brittle and prone to failure. System Verification is uniquely positioned to fill this gap by becoming a leader in QA for AI—helping companies ensure the stability and reliability of their AI solutions.
Second, the rise of large language models (LLMs) and tools like GitHub Copilot has introduced new challenges for software development. While these AI-driven coding assistants boost productivity, they also carry risks. Junior developers, for instance, might rely too heavily on code suggestions without fully understanding the implications for maintainability and quality. As QA experts, we see an opportunity to become thought leaders in managing these risks, advising clients on how to leverage these tools effectively without compromising code quality.
Building the Future QA Team
Of course, stepping into an emerging market brings its own set of challenges. We’re in uncharted territory, which makes hiring the right people tricky. You need the right skills, but you also need flexibility to adapt as client needs evolve. Rather than looking externally, we saw an opportunity to tap into our internal talent pool.
I began recruiting team members who had an interest in AI—some had dabbled in the field, even though it wasn’t part of their job description. Together, we’re pushing forward, developing technical solutions that will help System Verification become a key player in AI-focused QA.
Spreading the AI Knowledge
To drive AI knowledge company-wide, we’ve launched the "AI for Everyone" initiative. This includes lunch seminars, news sharing, and sponsoring employees to take the ISTQB AI Tester certification. Beyond the formal structure, our AI team is actively engaging with senior testers to spread our learnings. The idea is simple: empower senior testers to become AI ambassadors who can, in turn, mentor junior colleagues. This grassroots approach ensures a continuous flow of AI knowledge throughout the company.
Looking Ahead
In the near future, I envision System Verification becoming a trusted partner for clients who are grappling with the quality of their AI-generated code. As our testers grow more comfortable with AI tools, we’ll not only enhance our service offerings but also improve efficiency across the board. Long-term, it’s my hope that organizations using AI systems will recognize the need for robust QA—because, while AI may be complex, our commitment to quality will always be simple.