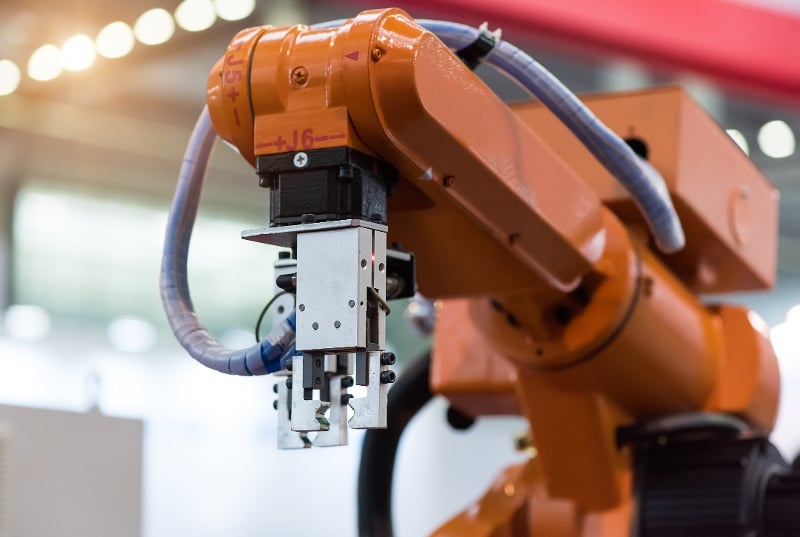
Key Benefits in Manufacturing and QA
- Cost Reduction In both domains, predictive maintenance helps reduce costs by allowing teams to focus on issues just before failure. For manufacturers, this means only replacing equipment when necessary. In software QA, it means addressing critical bugs and performance issues before they escalate into system-wide failures or outages.
- Minimized Unplanned Downtime For manufacturers, predictive maintenance prevents costly shutdowns. In software, the predictive approach minimizes unexpected system downtime by addressing vulnerabilities early, ensuring smooth operations, and enhancing system stability.
- Improved Resource Allocation Predictive analytics helps both manufacturing teams and software testers allocate resources more effectively. By targeting high-risk areas, maintenance and QA teams can focus on critical tasks without over-allocating time to routine or less urgent work.
- Increased Safety and Quality In manufacturing, predictive maintenance improves workplace safety by preventing catastrophic equipment failures. In software development, AI-powered QA ensures higher product quality by identifying and addressing defects or vulnerabilities before they affect the end user.
- Enhanced Efficiency and Performance Predictive analytics also ensures that manufacturing lines and software systems perform optimally. In manufacturing, this reduces production delays. In software, predictive models ensure that systems run efficiently under heavy loads, preventing performance degradation.
Predictive Maintenance in Software QA
AI can significantly improve the QA process by identifying failure points before they occur. Predictive models, for example, can monitor software usage patterns to detect vulnerabilities that might cause future downtime or performance issues. These models can also help optimize regression testing by selecting and prioritizing test cases most likely to identify critical bugs, improving overall software quality.
By integrating predictive maintenance into software QA workflows, companies can deliver more stable and reliable products to their customers while reducing the risk of critical defects causing unplanned system downtime.
Conclusion: The Future of Maintenance and QA
AI-powered predictive maintenance is transforming both manufacturing and software development. By adopting a proactive, data-driven approach, businesses can reduce the risks associated with downtime, improve operational efficiency, and deliver better quality products and services.
For companies seeking to minimize downtime, whether on the manufacturing floor or in software systems, predictive maintenance is not just a technological upgrade—it’s a strategic investment in reliability and quality.